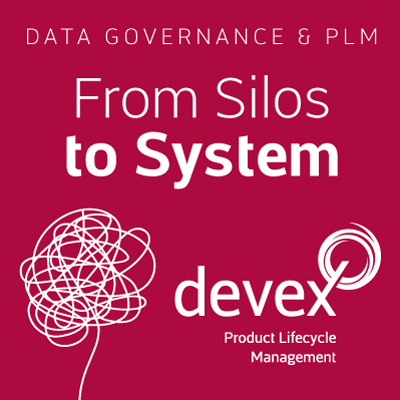
- Home
- PLM & Compliance Blog
- How to Take Data Governance from a Siloed Approach to a Systematic Process
How to Take Data Governance from a Siloed Approach to a Systematic Process
|
Product Lifecycle Management
|
Food & Beverage
Posted By:
Jenna Fu
From getting products to market and registered in multiple countries, to translating innovation requests into product launches and juggling the global costs of goods in a complex portfolio, process-based manufacturers aren’t just dealing with more data--they’re dealing with the need to have deeper connectivity between data systems. Implementing a comprehensive data governance plan across these initiatives can quickly turn into a trip to uncharted waters.
Why most modern data governance strategies fall short
Manufacturers typically desire the ability to implement more visibility and tighter control over these various interconnecting data streams; however, significant roadblocks exist for realizing this streamlined vision.
There’s often no centralized ownership over data governance efforts
Today, product data lives between multiple systems, such as ERP, CRM, PLM, and more. Even with tight integration, data originates and is managed by separate groups among the functional Innovation, Marketing, Regulatory teams as well as outside vendors and suppliers. Even if data fields between departments are the same, data requirements, security, regulatory standards and access points may not be. Conflicting or incongruent data usage between many different groups make straightforward data sharing and data harmonization difficult. It is usually not clear who should lead the charge in creating governance standards.
Data governance pain points are only uncovered during critical, time-intensive initiatives
The scale of poor data management often becomes a visible pain point only during crunch times. New project launches unearth the inaccessibility or inconsistencies of data between departments, making it difficult to capitalize on past projects learnings. Mergers or acquisitions expose system redundancies and the need for complex data consolidation or application decommissioning. Lack of data access across departments slows down critical regulatory approval timelines. While these initiatives expose the problem, these projects include too many other high-priority tasks to spare resources on solving the underlying lack of data governance standards.
Creating a data governance team includes initial upfront costs
Even when data governance is identified as a critical initiative, forming and hiring a dedicated data governance team can internally be a hard sell. The additional headcount needs to be reconciled with the time costs and process inefficiencies that poor data governance creates throughout the business. Calculating the effect that inefficient data governance has on product development timelines and regulatory delays is not always a straightforward proposition due to the cross-departmental nature of data management. With fluctuating margins and cost of goods, it’s very difficult for most manufacturers to bite the bullet and incur additional costs to create a data governance department or group.
Standardizing data governance efforts at every PLM stage
There’s no one-size-fits-all solution for controlling data governance. When translating governance standards into data design and system integration, manufacturers should be encouraged to think holistically across processes, systems and each department’s strategic initiatives.
Set systematic data validations and approvals
For each department or project type, it’s critical to first identify the data owners and managers of each information type—and the primary purpose of that data. Those decisions can be used to guide automated data validation limits and approval steps. For instance, in order to meet certain regulatory standards for raw material or ingredient amounts, product developers may want to ensure that a specific limit on ingredient percentages is not exceeded within the formulation. This analysis example first needs the ingredient information captured, and then can be enforced within a PLM system’s data fields to automate the governance of that ingredient data.
Define security roles and access rights for different groups
When data validations are being determined, it’s also advisable to appoint a data governance representative from each team or department. Creating a council of data champions, or least assigning a point person within a department to own data management, will help keep governance standards in line with top corporate initiatives.This also helps resolve competing interests that affect data security and data access. As more internal systems move to cloud-based, SaaS solutions that offer deeply connected process automation and data analytics options, manufacturers will need to weigh the effect of transparency against security needs. For data fields that cross departments and drive collaboration steps, determining the right level of data access is critical to protecting proprietary product data while still enabling each group to efficiently complete daily and strategic work.
Set data harmonization parameters
Once there is a clear sense of which data crosses departmental and system boundaries and its primary use, harmonizing data between departments and systems often then becomes a tug-of-war between data requirements. In order to avoid this situation, it is best to first attempt to identify extraneous or legacy systems where data is replicated, and then reduce the number of instances of the same data.
Based on your business needs, it’s important to select a master system as the primary source of data. If no overlap in data values exist, use decision tables to prioritize the data formats. For some organizations, the PLM system will serve as the master system single source of the truth. For others, it is the ERP system that serves in this capacity, with the PLM system integrating into it.
Want to learn more? Read our post "Best Practices for Harmonizing Global Product Data"
Implement workflows that systematically enforce data collection
Agreeing on a data structure makes it easier to then add an enterprise-wide automation layer into the framework to enforce those standards, rather than trying to stitch together existing workflows, data fields and integration points.
When considering system design in a PLM, CRM or ERP system, data governance teams should also think ahead and plan out specific KPIs that the company needs to support short and long-term objectives that drive data capture. For example, if a company is focused on a cost-saving initiative, tracking formulation costs at the material level could be facilitated by a workflow that does not allow the developer to proceed forward without entering in a material cost. A workflow that is enforced within the system across all groups ensures that those data fields are completed in a timely manner for easy assessment toward achieving company objectives.
Using data governance to drive trend analysis
When proper data standards are implemented and enforced throughout PLM processes, the accuracy of that data and enterprise-wide data access can be used to support strategic initiatives such as the following three areas:
Conducting trend analysis across the product portfolio
Without extraneous systems and data silos to sift through for trend analysis, innovation teams can better understand which product types and ingredients resonate best with consumers across a broader range of data points. Strategic reporting dashboards can then be created that provide real-time portfolio breakdowns by category, market, brand, number of projects by stage and many more. Centralizing data governance standards allows manufacturers to see a much wider range of views into business operations, providing a significant competitive edge.
Tracking financial targets for innovation teams
By integrating financial ERP data with product data applying the same governance standards, manufacturers can enable product developers to see the full availability of current or experimental ingredients across the product portfolio. This enables them to select the most cost-effective materials at the beginning of the innovation process. With product data and costs tracked efficiently across the product lifecycle, financial teams can better analyze the potential impact of new or changing innovation ideas on the bottom line, down to the raw material level.
Identifying areas for product improvement or market growth
Standardizing all specifications, recipes and data fields in one place, preferably a PLM system, benefits the whole company. By centralizing global product sales, manufacturing costs, supplier and vendor data in one place in a standardized, agreed-upon, compliant format, manufacturers can gain a more comprehensive snapshot of product attributes, risks and costs. Modern data governance is really about being able to trace elements in multi-layer formulas for regulatory requirements that affect easily substitutable ingredients across global formulas to meet requirements for selling across multiple markets.
Implementing these strategies and outcomes may seem difficult for global manufacturers with complex global product lines. But, a robust PLM system help ease the burden by automating enterprise standards within product data and system design. In the age of the digital product, maintaining disjointed siloed data with nominal, ad-hoc governance strategies is a recipe for falling significantly behind the competitive curve. When manufacturers take the time to update governance strategies to match today’s interconnected system design, innovation, collaboration and market growth will flourish and lead to growth and competitive advantage.